- July 19, 2022
- Posted by: sadmin
- Categories: Customers
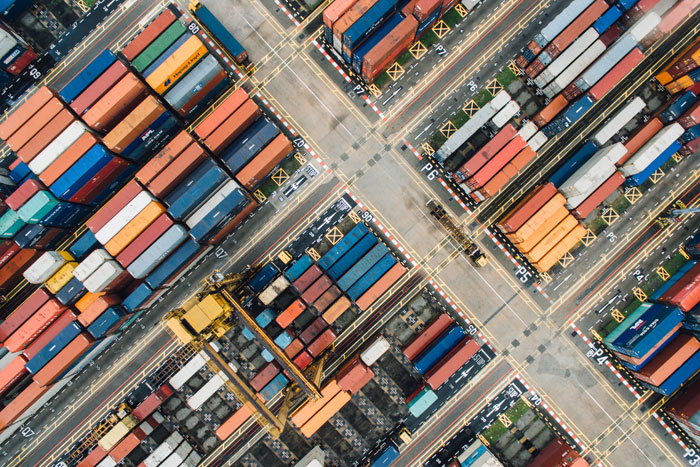
Given the recent significant worldwide disruptions brought on by the pandemic, supply chains have witnessed an increased requirement for resilience. To improve its current operations, the sector has responded by turning to cutting-edge technologies, such as artificial intelligence and machine learning.
Every supply chain step uses AI to increase productivity, lessen the effects of a global labor shortage, and develop safer and more intelligent ways to get products into customers’ hands. Manufacturing, storage, and distribution are all areas where AI is being used. According to McKinsey & Co.’s research, supply chain managers that implemented AI in their operations witnessed more than 60% reduction in costs.
According to that same survey, most supply chain management responders are likely to identify savings mainly attributable to spend analytics and logistics-network optimization. It is undeniable that AI and machine learning are revolutionizing several industries, notably supply chain and logistics.
The following six use scenarios demonstrate how decision-makers might arrive at a more effective and economical method of handling, storing, and moving items.
Demand forecasting:
According to a McKinsey poll, 80% of supply chain executives either expect to use AI/ML in planning or are already doing so. This is positive because reliable and effective supply chain management depends on accurate demand forecasting. With the proper implementation, supply chain managers can adequately forecast and spot changes in upcoming consumer demand.
Supply chain managers can then do strategic business and purchasing decisions when planning inventory, without producing a surplus or understocking, by drawing on the current supply chain process and software data. Given the increased pricing and decreased inventory stockout that accompany accurate demand forecasts, this, in turn, increases revenue.
Warehouse management:
By streamlining the movement of goods into and out of the warehouse, ML helps with warehouse management. Warehouse managers can effectively utilize the available warehouse space by using predictive models. Employees, such as product pickers, can work more efficiently and effectively when the warehouse is well-organized, which increases their capacity for order fulfillment.
Beyond raising employee productivity and streamlining order fulfillment, optimal warehouse space has further advantages. Supply chain managers can buy items in large quantities according to the increased storage capacity of warehouses, thanks to their optimized space utilization. Bulk purchases reduce expenses and increase profit margins because they are less expensive.
Inventory management:
Proper inventory management is essential since omnichannel delivery is a high priority for 94% of businesses. Supply chain managers may monitor inventory control and stock levels in real-time by integrating AI into existing software architecture and data lakes. An integrated AI/ML system can forecast the amount of stock required based on the situation if the correct data is sent into it.
For instance, a material scarcity could result in lower manufacturing of a particular product. This enables supply chain managers to precisely forecast the number of goods in their inventory to satisfy consumer demand. This is useful for organizing inventory supply and making business decisions based on data to prevent overstocking or understocking.
Fleet management, route optimization:
To manage your fleet, make data-driven decisions based on information received from traffic, weather, and other external factors. Fleet managers may choose the most efficient routes to get their fleets to their destinations on time with the help of pertinent information and precise data insights.
Fleet operators can alter routes in real-time by combining ML with information gathered by IoT devices and sensors onboard fleets. Making route decisions based on current weather and road conditions enhances driver and vehicle safety. An adequately managed fleet will have positive downstream consequences such as improved customer service and productivity.
Predictive maintenance:
Consider a supply chain workflow that operates smoothly (like it should!). Now picture one piece of equipment failing suddenly, followed by others over a few months. Unplanned maintenance schedules cause delays and decreased productivity throughout the whole supply chain. The key to creating a seamless end-to-end process has equipment that is consistently up and operating.
Advanced analytics can boost up to 20% equipment uptime by predicting breakdowns. Supply chains can use a predictive maintenance strategy to keep their equipment in good working order and free from unforeseen breakdowns. A predictive maintenance plan enables supply chains to anticipate machinery breakdown with AI and advanced analytics.
Conclusion:
You now have it. These are the most typical problems and use cases that AI/ML can resolve. An investment in AI can help you achieve a competitive edge by resulting in considerable cost savings and efficiency gains that will enable you to satisfy expanding client needs better. To implement an ML strategy, you must have the infrastructure, data storage, and collecting capabilities.
Companies can start enjoying the benefits of ML sooner the earlier they start planning. A growing number of AI/ML suppliers are making designing and deploying models simpler and more accessible than ever to get started with the technology. This is a crucial success element for all supply chain phases.