- June 14, 2022
- Posted by: sadmin
- Categories: Customers
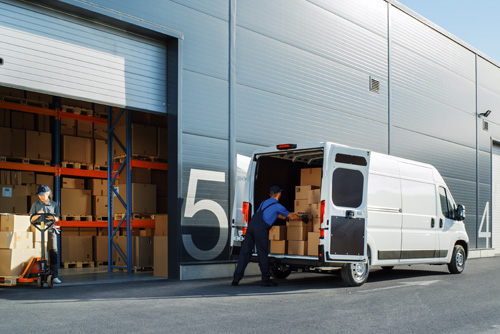
Firms’ procedures to acquire insight and extract value from the massive amounts of data related to the purchase, processing, and delivery of goods are called supply chain analytics. The use of supply chain analytics is an integral part of supply chain management (SCM). Although supply chain analytics has been there for over a century, different mathematical models, advanced data infrastructure, and modern applications that support it have progressed substantially.
Mathematical models have improved with better statistical techniques, predictive modeling, and machine learning. Cloud infrastructure, complex event processing (CEP), and the internet of things have all revolutionized data infrastructure. Traditional application silos like ERP, warehouse management, logistics, and enterprise asset management have grown to provide visibility across them.
One of the important reasons to use supply chain analytics software is to increase forecasting and efficiency and be more responsive to consumer demands. Predictive analytics using point-of-sale terminal data saved in a demand signal repository, for example, can help a company anticipate consumer demand, leading to inventory cost savings and speedier delivery.
End-to-end supply chain analytics facilitates the integration of data starting with raw material acquisition and continuing through production, distribution, and aftermarket services. This is contingent on successful integration across the numerous SCM and supply chain execution platforms that make up the supply chain of a typical organization. Such integration is supply chain visibility, or the capacity to see data about commodities at every stage of the process.
Supply chain analytics software
Supply chain analytics software comes in two flavors: integrated within supply chain software or as standalone business intelligence and analytics tool with access to supply chain data. Most ERP providers, as well as vendors of specialized SCM software, offer supply chain analytics capabilities. Software models that may be adapted and incorporated into a company’s business operations are developed by some IT consultancies.
Some ERP and SCM companies have begun to integrate CEP into their platforms for real-time supply chain analytics. One-to-one interfaces are available from most ERP and SCM providers, but there is no standard. The Supply Chain Operations Reference (SCOR) model, on the other hand, provides standard criteria for evaluating supply chain performance against industry benchmarks.
How does supply chain analytics work?
Supply chain analytics integrates data from many applications, infrastructure, third-party sources, and emerging technologies such as IoT to improve decision-making throughout strategic, tactical, and operational operations of supply chain management. Supply chain analytics enhances real-time visibility into supply chain activities and their impact on customers and the bottom line of the business, which helps to synchronize supply chain planning and execution.
The improved visibility can also administer decision-makers in better analyzing costs against customer service tradeoffs, resulting in more flexibility in the supply chain network. Creating supply chain analytics usually begins with data scientists who are familiar with a particular component of the organization, such as cash flow, inventory, waste, and service levels.
Data engineers deploy various supply chain analytics models that meet a specific level of success in production with a focus on scalability and performance. Data scientists, data engineers, and business users collaborate to improve the presentation and operationalization of data analytics in practice. Correlating the efficacy of data analysis models in production with the business value they bring improves supply chain models over time.
Features of supply chain analytics
Supply chain analytics software usually includes most of the following features:
Data visualization: Data visualization is the capacity to slice and dice data from many perspectives to better understand it.
Stream processing: Stream processing is extracting information from many data streams supplied by various sources such as the Internet of Things, applications, weather reports, and third-party data.
Natural language processing: Extracting and organizing unstructured data hidden in papers, news sources, and data feeds using natural language processing.
Location intelligence: Location intelligence is the process of extracting information from location data to better understand and improve distribution.
The digital twin of the supply chain: To improve predictive and prescriptive analytics, a digital twin of the supply chain is created by organizing data into a comprehensive supply chain model shared among different types of users.
The application of supply chain analytics
Supply chain analytics is used in sales and operations planning to match a manufacturer’s supply with demand by developing plans integrating daily operations with corporate strategy.
The following are some of the other things supply chain analytics is used for:
- Improve risk management by recognizing known hazards and forecasting future risks based on supply chain patterns and trends;
- Improve planning precision by examining customer data to identify factors that cause demand to rise or fall;
- Consolidate data sources to assess inventory levels, estimate demand, and identify fulfillment difficulties;
- Enhance order management by consolidating data sources to assess inventory levels, predict demand, and identify fulfillment issues;
- Improve contract negotiations and discover chances for savings or alternative suppliers by streamlining procurement by coordinating and analyzing spending across departments;
- Improve models for evaluating inventory levels required to meet service goals with little capital expenditure to boost working capital.
Future trends of supply chain analytics
Supply chain analytics will expand in lockstep with analytics models, data structures, technology, and the capacity to combine data across application silos. Advanced analytics will, in the long term, lead to more autonomous supply chains that can manage and respond to changes, much like self-driving cars are doing now.
Improvements in IoT, CEP, and streaming architectures will also allow businesses to gain insight from a broader range of data sources quickly. People’s capacity to create more accurate and relevant predictive insights that can be implemented into workflows will continue to increase as AI develops.
The following technologies are likely to play a significant role in supply chain analytics and management:
Blockchain: Blockchain infrastructure and technology have the potential to improve visibility and traceability across the supply chain at a higher level. Companies may be compelled to employ smart contracts to automate, control, and execute transactions due to these similar building blocks.
Analytical graphs: Within a decade, graph analytics is expected to power more than half of all enterprise applications, allowing supply chain managers to better evaluate the links between various supply chain entities.
Hyper-automation: The technology that enables hyper-automation will speed up supply chain automation by identifying automation candidates, generating automation, and managing these automated operations using process mining analytics.