THE PROBLEM
Machine breakdowns in the manufacturing process introduce downtimes. The client was making use of dashboards to keep track of several statistics and metrics about product rejections and machine breakdowns. However, these dashboardsentailed intricate manual monitoring, and yet were not adequate in enabling the clientautomatically to identify anomalous trends and patterns in product rejections that might warrant specific interventions in the process. In addition, the client wanted to detect anomalies in machine breakdown patterns for identifying predictive maintenance requirements. Hence, the client collaborated with Inxite Outto develop a mechanism for automated detection of anomalous trends in key product rejection metrics and machine breakdown anomalies in an unsupervised manner, to predict and prevent unplanned stops and breakdowns when possible.
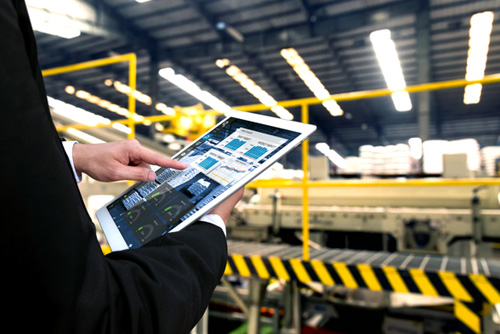
INXITE OUT APPROACH
Data Cleaning
Explored historical data to identify data quality issues in product rejections and machine breakdowns records including incorrect time overlaps, out of range data etc., and worked with the business stakeholder to align on strategies to clean the data before performing any further analysis.
Product Rejection Anomaly Detection
Used AI models to perform univariate and multivariate anomaly detection on patterns of product rejection volumes for any given machine in relation with machine manufacturing volumes, in unsupervised and automated fashion. The output data contained periods of anomalies found during user-specified time windows,along with the underlying reasons, for easy consumption, visualization, and further action.
Machine Breakdown Anomaly Detection
Developed statistical models to identify significant trends in machine breakdowns, and to detect sudden changes compared to historical patterns, on a level of granularity of aggregation in alignment with the business.
RESULT
Post completion of POC a pilot was run on the 2 largest plants in the country of operation. The solution was able to detect anomalies along with the reasons explaining the anomalies – which were manually validated by the client’s team of experts. Success of the pilot led to the solution being undertaken for global roll out